In the modern, data-driven world, businesses are producing and utilizing vast volumes of data from innumerable sources. However, unless information is appropriately gathered, combined, and examined, raw data is useless on its own. By incorporating artificial intelligence (AI), this process—which has historically been time-consuming and prone to human error—has seen revolutionary breakthroughs. World BI is organizing Clinical Trials Innovation Programme again where this topic is going to be discussed. Through the automation and optimization of all phases—collection, integration, and analysis—AI is assisting companies in transforming massive amounts of data into insightful knowledge. Let's examine how artificial intelligence (AI) is simplifying these fundamental data operations and changing sectors as they go.
AI-Driven Data Collection: Smarter, Faster, and More Accurate
1. Automated Data Harvesting
Continuous and real-time data collection from a variety of digital sources, including as websites, sensors, APIs, and databases, is possible with AI-powered bots and crawlers. Implementing automated data capture mechanisms reduces manual effort and minimizes errors. For instance, using barcode scanners in a warehouse to track inventory levels ensures real-time updates without human intervention.
For example:
- E-commerce platforms collect customer behavior data using AI-based tracking.
- Healthcare systems extract patient data from wearable devices via smart algorithms.
Unlike manual collection, these systems are tireless and highly scalable, reducing the risk of missing or inconsistent data.
2. Natural Language Processing (NLP) for Unstructured Data
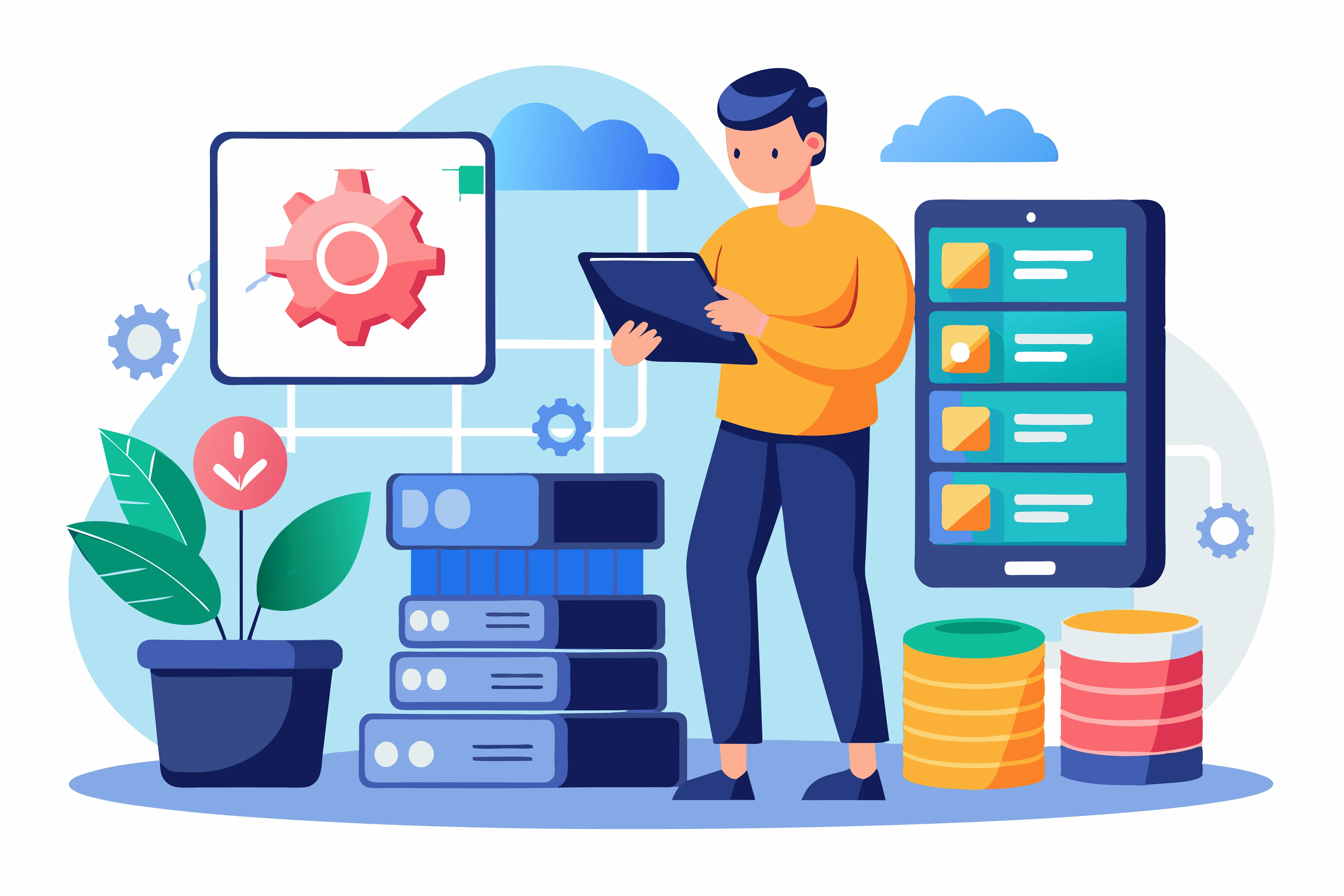
One of the biggest challenges in data collection is handling unstructured data (emails, social media, PDFs, chat logs). AI, especially NLP, can now process and extract key information from such formats:
- Analyzing customer feedback from reviews and tweets.
- Summarizing legal documents or contracts.
3. Real-Time Data Streams
AI makes it possible to import data in real time from financial markets, logistics systems, and Internet of Things sensors. This is especially helpful in fields like:
- Manufacturing, where sensor data helps in predictive maintenance.
- Banking, where real-time fraud detection relies on instant data analysis.
Data Integration: Making Disparate Systems Talk
Once data is collected, it often resides in silos—CRM, ERP, legacy systems, cloud platforms. AI simplifies the complex task of integrating and harmonizing these diverse sources.
1. Smart Data Mapping
AI algorithms can recognize similar fields across different systems and automatically map them, eliminating manual effort. For instance:
- Mapping “cust_id” in one system to “customerID” in another.
- Resolving inconsistencies in date formats or naming conventions.
2. Data Cleaning and Deduplication
Dirty data costs companies millions. Compared to conventional rule-based systems, AI is significantly more effective at scanning for outliers, duplication, and abnormalities.
- Identifies mismatches and automatically corrects or flags them.
- Uses machine learning to improve over time as it learns from historical corrections.
3. Data Enrichment
AI tools can automatically augment internal data with external sources—adding demographics, social signals, or market trends—enhancing context and value.
For example:
- Enriching customer profiles with their public social media activity.
- Enhancing product listings with AI-curated descriptions from online reviews.
Data Analysis: From Raw Numbers to Strategic Intelligence
AI elevates analysis to a new level with sophisticated capabilities that standard BI tools just cannot match if clean, consistent data is available.
1. Predictive Analytics
AI models can uncover patterns that predict future behavior:
- Retail: Predicting customer churn based on shopping habits.
- Healthcare: Forecasting disease progression based on patient history.
These models improve with time, providing increasingly accurate insights.
2. Prescriptive Analytics
Going a step further than prediction, AI can recommend actions:
- Suggesting inventory restocks based on supply chain delays.
- Offering marketing strategies tailored to customer segments.
This enables data-driven decision-making at a strategic level.
3. Natural Language Generation (NLG)
AI can now generate textual reports from data:
- "Sales grew by 15% in Q2, with the highest performance in the Southeast region."
This eliminates the need for data analysts to manually write interpretations, making insights more accessible to non-technical stakeholders.
Real-World Use Cases
Healthcare
AI assists in combining medical records from labs, wearable technology, and hospitals, then evaluates them to suggest early disease identification and therapy.
Retail
Retailers use AI to gather customer data from web, app, and in-store activity, integrate it with purchase history, and generate personalized product suggestions.
Manufacturing
In order to forecast malfunctions and improve supply chain logistics, machine learning algorithms examine sensor data from several production lines.
Finance
Banks use AI for real-time data monitoring, fraud detection, regulatory compliance, and customer segmentation.
Challenges and Considerations
While AI brings enormous potential, it also comes with challenges:
- Data Privacy: AI systems must comply with regulations like GDPR and HIPAA.
- Bias in Algorithms: Poor data inputs can lead to biased decisions—regular audits are essential.
- Infrastructure Needs: Robust cloud or on-premise infrastructure is required to support AI workloads.
The Future of AI-Powered Data Ecosystems
We are moving toward a world where:
- Data is collected passively and securely.
- Systems are interconnected through intelligent data fabrics.
- AI-driven insights are delivered in real-time to decision-makers.
Technologies like AutoML (Automated Machine Learning) and AI-powered data lakes are set to further democratize data usage, making it easier for organizations of all sizes to leverage complex analytics.
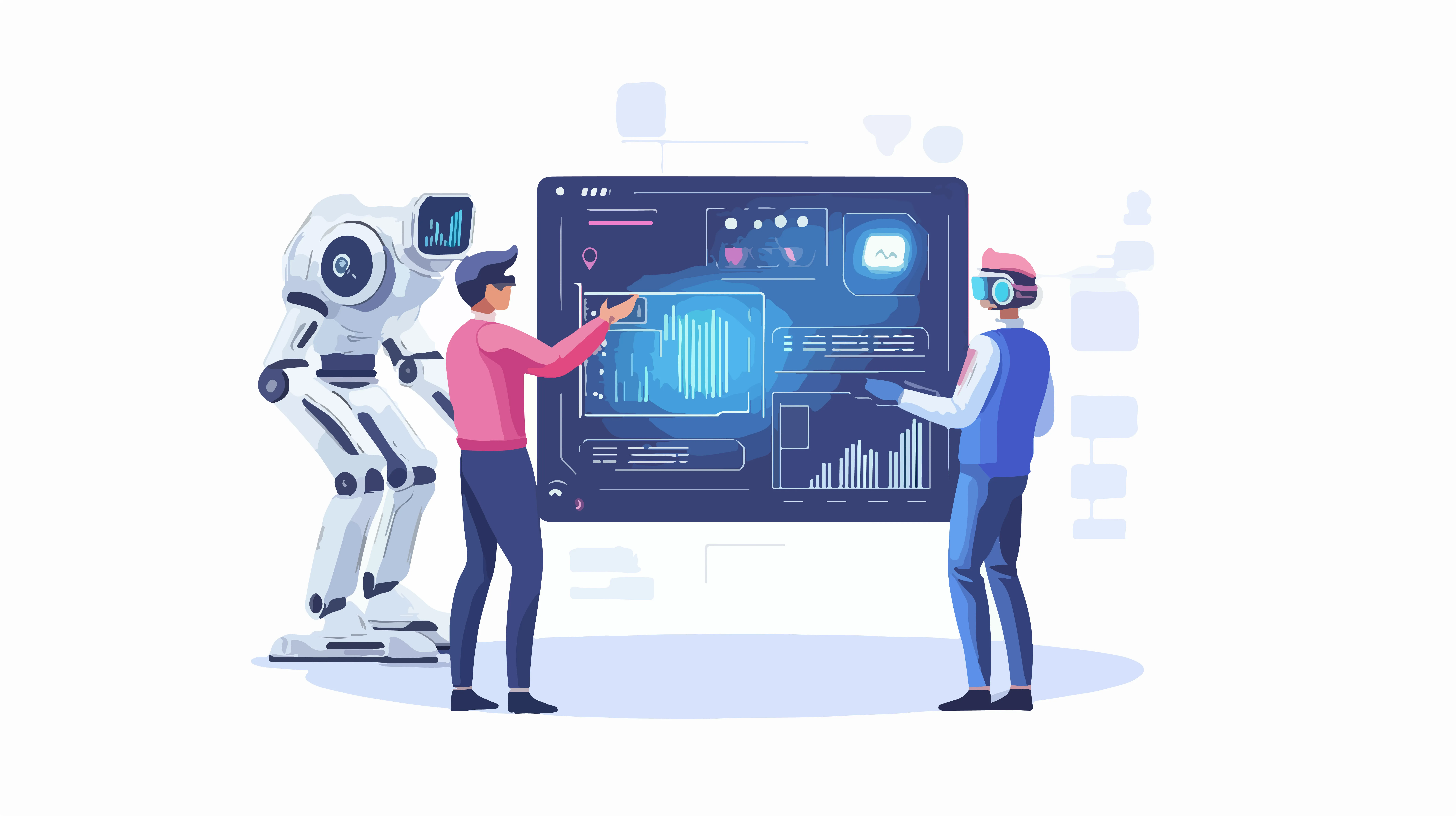
Conclusion
Artificial intelligence has evolved from a tool to the foundation of contemporary data tactics. Businesses may make quicker, more intelligent, and more assured decisions by using AI to automate and improve the data collecting, integration, and analysis processes. By adopting this change, businesses will be able to extract enormous value from their data and obtain a competitive advantage in their respective markets. Our current data handling practices set the stage for the intelligent future.
Clinical Trials Innovation Programme
Clinical Trials Innovation Programme is a global event uniting leading pharmaceutical, biotech, and clinical research organizations along with AI, data science, regulatory, and clinical operations experts to explore the future of clinical development. Clinical Trials Innovation Programme organized by World BI, this dynamic conference focuses on clinical trial design, patient recruitment, operations, decentralized trials, real-world data, regulatory compliance, and AI-driven innovations in clinical research. The event fosters cross-industry collaboration and innovation to enhance the efficiency, diversity, and success of clinical trials globally.