Developing medications for uncommon disorders requires a careful strategy that balances market access and regulatory requirements while ensuring patient safety. Limited patient populations, diverse illness presentations, and lack of historical data make rare diseases challenging for biopharma firms and clinical research site personnel. Traditional randomized control trials often fail in these settings, necessitating creative methods to collect useful data and ensure patient safety.
World BI provide a platform in Clinical Trials Innovation Programme to provide best solution in managing the trials data of rare diseases. It provides the opportunity to select the best service for your clinical trials design.
Clinical Data Management:
- Clinical data management (CDM) is a crucial aspect of clinical research, ensuring the accuracy and reliability of data gathered during testing.
- It involves procedures like data collection, cleansing, and administration, aiming to produce high-quality data.
- The first step is collecting information from clinical trials participants.
- The information includes medical background, treatment, and results, from various sources like surveys and electronic medical records.
Safety Factors in Trials For Uncommon Diseases:
- Biopharmaceutical businesses need to implement specialized risk management techniques to handle these complications.
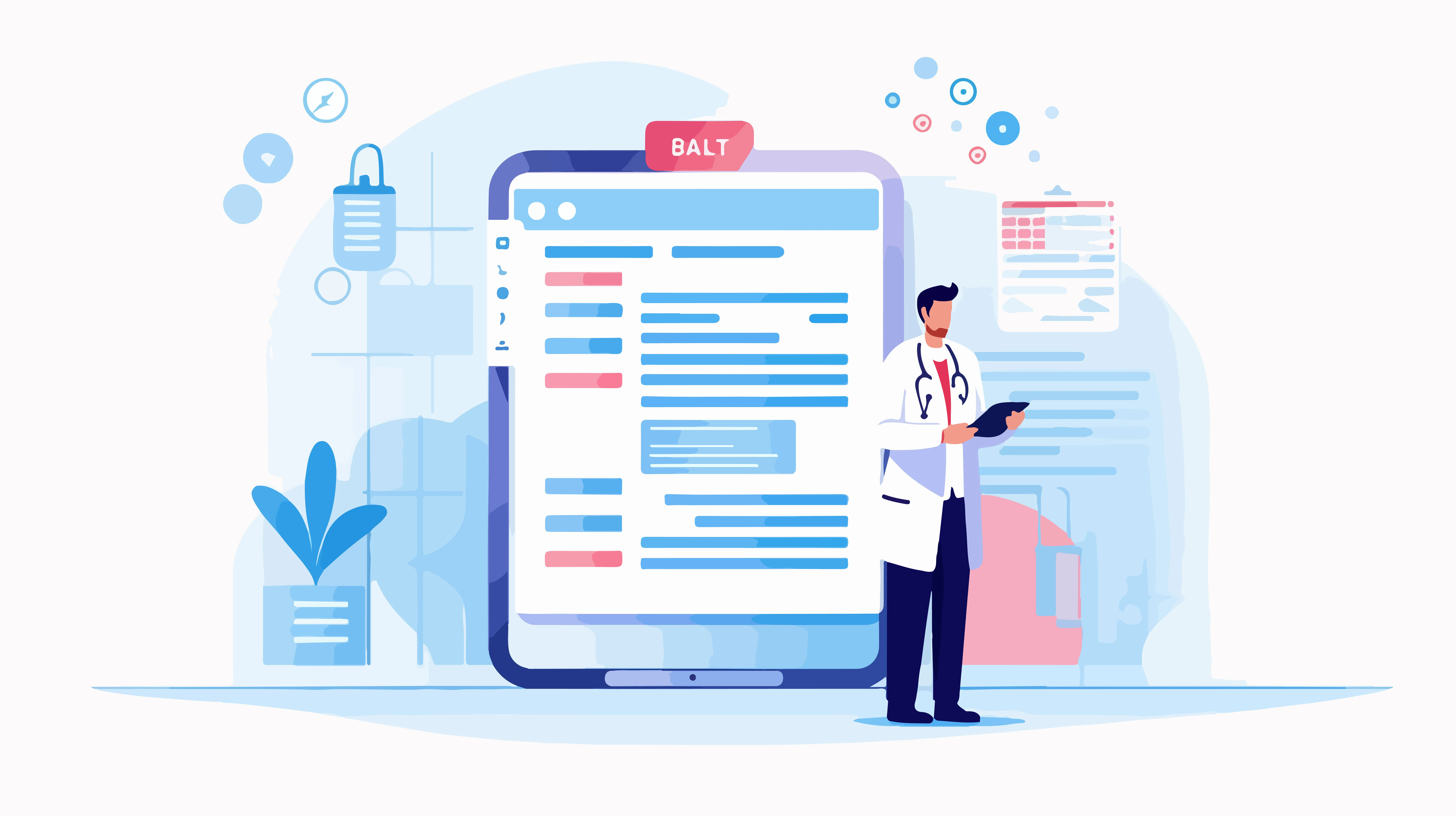
- This entails anticipating possible hazards, creating thorough strategies for mitigating them, and regularly modifying these plans in light of new information.
- Strong patient monitoring procedures are necessary.
Clinical Data Management Process:
Data Collection:
- The first step is collecting information from clinical trials participants.
- The information includes medical background, treatment, and results, from various sources like surveys and electronic medical records.
- The information is collected from various sources like questionnaires, lab tests, and electronic health records.
Data Cleaning:
- The data undergoes a cleaning process to identify and correct errors, ensuring accuracy and readiness for statistical analysis.
Organizing and Securing:
- Data management involves securely organizing and storing the cleaned data, adhering to regulatory standards to protect participants' privacy and ensure data integrity.
Strategies:
1. Prompt and Thorough Planning:
Customized DMP (Data Management Plan):
- Create a thorough DMP that describes the procedures for gathering, tracking, and analyzing data.
-
The DMP should meet particular demands, such as the following, given the complexity of rare illness trials:
- Features of rare diseases (patient population heterogeneity).
- Difficulties brought on by tiny sample numbers.
- Standardization of data for populations and places that may differ.
Unambiguous Protocol Design:
- To direct data collection and reduce uncertainty, make sure the clinical trials protocol is well-defined, particularly with relation to outcome measures.
2. The Utilization of Electronic and Centralized Data Collection Methods:
Electronic Data Capture (EDC):
- EDC solutions can help to increase data accuracy, decrease mistakes, and speed data collecting.
- Additionally, EDC systems can provide real-time data monitoring, which is essential for rare illness studies where each and every piece of information is important.
Mobile Instruments for Gathering Data:
- Consider using wearable technology or smartphone applications to capture real-time patient data, especially for remote or geographically dispersed patients.
Patient Reported Outcomes:
- In order to better understand the patient's experience with the condition, rare disease studies frequently call for the collection of patient-reported outcomes, or PROs.
- Utilize electronic versions of verified PRO equipment to guarantee consistency and data input simplicity.
3. Early Stakeholder Engagement:
- Early planning should involve key stakeholders—clinical operations, investigators, data scientists, statisticians, and regulatory affairs—to ensure data management aligns with trial goals.
- Collaboration ensures data management plans complement the overall trial objectives.
- Communication Plans: Provide unambiguous avenues for reporting problems, developments, and advancements.
4. Effective Integration and Exchange of Data:
- A variety of sources, including different locations, labs, imaging centers, and patient-reported outcomes, provide data for clinical studies.
- All of this data will be correctly matched and able to be examined holistically with the help of an efficient data integration approach.
- Centralized Database: To instantly compile data from several sources, use a centralized database.
- Interoperability: Make sure that systems (such as imaging, laboratory information, and EDC systems) that are used to gather, store, and analyze data can readily interface with one another.
- Collaboration and Data Sharing: Establish channels for safe, regulated data exchange amongst clinical trials participants, such as statisticians, data scientists, researchers, and regulatory bodies.
5. Pay Attention to Documentation and Regulatory Compliance:
Submission to Regulation:
- Assure adherence to regulatory directives, such as ICH E6, 21 CFR Part 11 (FDA), and regional clinical trials laws.
- Preserve audit trails, safe data storage, and unambiguous records of data management practices.
Regulations for Orphan Drugs:
- Utilize rare disease-specific regulatory channels like expanded access, accelerated approval, and orphan drug designations, which have unique data needs.
- Ensure data management aligns with these requirements.
6. Data Integrity and Standardization:
An essential component of a successful clinical study is standardizing the data and guaranteeing its integrity. This comprises:
Data Standards:
- To make integration, analysis, and reporting to regulatory bodies simpler, use industry-standard data formats (such as CDISC standards for clinical data).
CRF Design:
- Create case report forms (CRFs) in accordance with the research protocol, making sure that the necessary data is recorded in an organized and consistent way.
Validation of Source Data:
- Make sure the information in the CRFs can be linked back to the original source data (such as lab results or medical records), and do regular reconciliation to look for inconsistencies.
Audit Trails:
- Keep thorough audit trails that record every modification made to the data, along with the time and identity of the alteration.
- This is crucial for regulatory compliance and data transparency.
7. Data Quality and Validation:
- High-quality data is crucial for clinical studies, resulting in reliable outcomes.
- Validation checks during data entry identify errors early, saving time.
- Regular audits compare data to original sources, ensuring accuracy.
- Encouraging employee education on optimal practices and promoting candid communication helps address concerns.
- These measures ensure data input and reporting are accurate and reliable.
8. Patient-Centric Data Management:
- Develop strategies to keep patients engaged in the study, including regular communication, support services, and addressing patient concerns.
- This also helps with minimizing dropouts and maintaining a complete dataset.
9. Enhance Patient Safety:
Timely Safety Monitoring:
- Prompt reporting, tracking, and monitoring of adverse events (AEs) and serious adverse events (SAEs) are guaranteed by efficient data management.
Integrity of Data in Safety Reports:
- Post-trial monitoring and regulatory filing depend on timely and accurate safety data.
- In safety reporting, maintaining data integrity helps avoid missing possible hazards or negative consequences.
10. Data Privacy and Security:
- Make sure that patient data is safeguarded by robust encryption.
- Respect international regulations like GDPR or HIPAA to protect patient privacy.
- When sharing data for study, publishing, or regulatory submission, use anonymization procedures to safeguard patient identity.
Advancements in Clinical Data Management:
- Clinical data management has evolved significantly in recent years.
- EDC systems enable real-time data input, expediting procedures and reducing errors.
- Cloud technologies provide safe storage options, allowing researchers to access data anytime and anywhere.
- Hence improving teamwork among geographically dispersed groups.
- Mobile applications enable patients to input health information, while wearable technology tracks patient parameters, providing crucial health information.
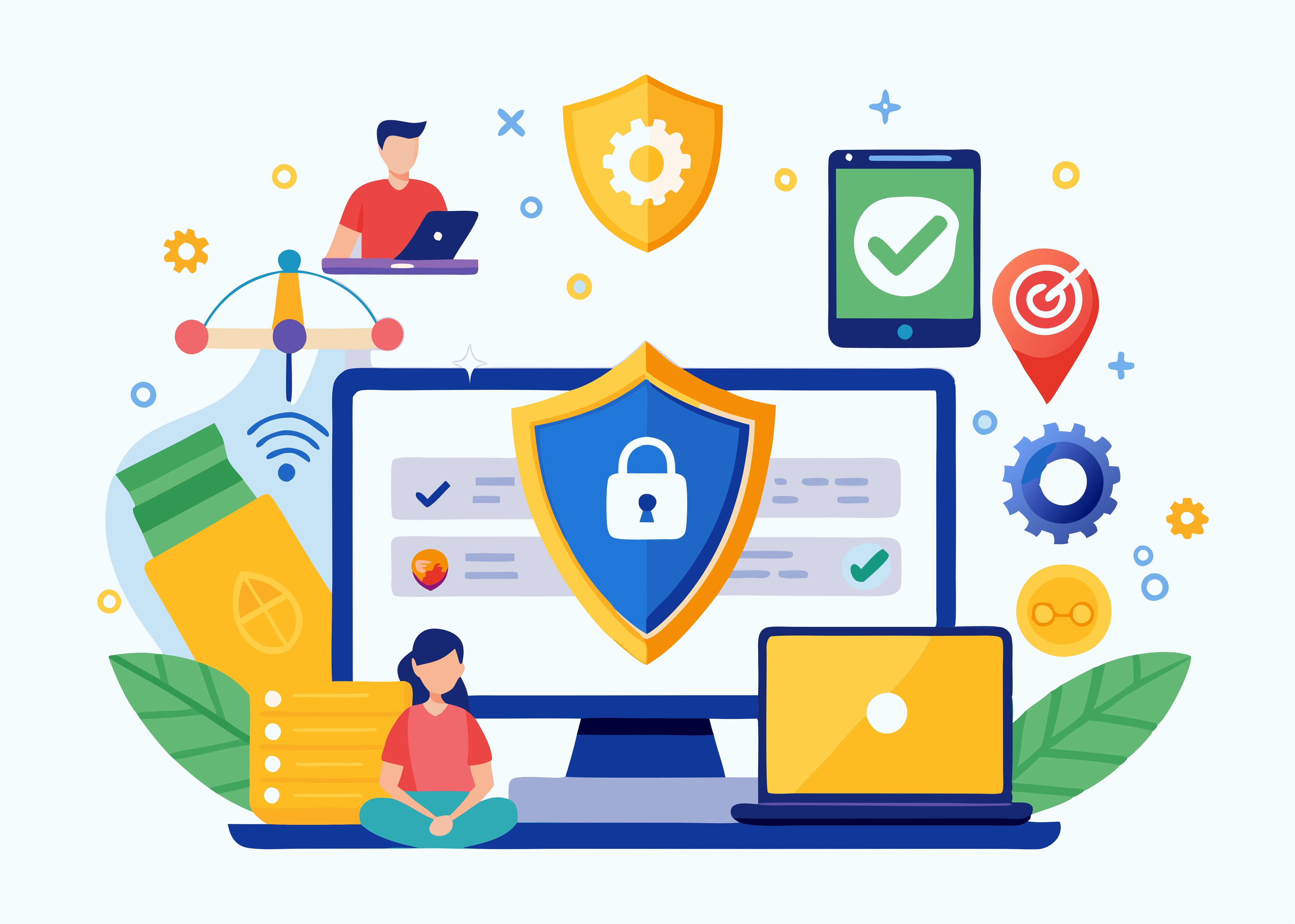
Applying Artificial Intelligence to Data Management:
- Artificial intelligence (AI) is revolutionizing clinical data management by enabling rapid evaluation of large data sets, identifying trends, and speeding up research.
- AI excels in predictive analytics, aiding in resource allocation and experiment arrangement and aiding in data validation and cleansing.
Conclusion:
- Clinical data management is crucial for successful trials, ensuring data integrity, security, and compliance.
- Understanding roles, tools, and best practices can enhance research outcomes.
- Staying informed about trends and staying informed about resources can boost efficiency and build trust with stakeholders.
Clinical Trials Innovation Programme:
A robust data management strategy not only enhances the scientific rigor of the trial but also fosters trust with regulators, stakeholders, and patients, ultimately leading to more successful outcomes and better treatment options for those suffering from rare diseases.
This opportunity will be offered through the Clinical Trials Innovation Program, which is organized by World BI and gives renowned pharmaceutical companies a forum to exchange ideas and experiences. This helps to generate provide best solution for data management that can result in significant improvements in treatment options for patients with uncommon conditions.