Respecting the individual’s values!
The ability to increase estimate precision, and stratification is frequently employed in randomized clinical studies. The terms stratified allocation and stratified analysis are denoted by the two types of stratification: pre-stratification and post-stratification. To put it another way, pre-stratification is the random assignment of treatments to patients in each stratum, and post-stratification is total randomization. A primary difference between the two is that whereas post-stratification maintains the allocation ratio within each stratum merely on average, pre-stratification aims to preserve the treatment allocation ratio within each stratum.
Within clinical trials, patient stratification is an essential procedure that improves the accuracy and efficiency of medical research. Through the use of separate subgroups based on variables like genetic information, illness risk factors, or anticipated treatment responses, researchers may create more focused and effective studies. By bringing together professionals from the sector to exchange ideas and information, the World BI Clinical Trials Innovation Programme provides a forum for debating the latest developments and obstacles in this field.
Is Patient stratification is Essential for Clinical Trials?
- To expedite recruitment and provide a research population that is representative of the general population, clinical trial designers primarily permit a variable number of individuals per stratum. We only look at a linear model analysis of the data in order to investigate the improvement in estimator precision brought about by stratification.
- Stratification might not be able to lower variability in nonlinear models even in the absence of sampling error. On the other hand, the authors demonstrate that stratification boosts power even in the presence of increased variation, whereas in linear models, it is often known that power declines with increasing variance.
- In clinical trials, there are many particular variants in the ways that may be used to assign patients to treatment arms; these variations can be classified as belonging to one of the three main strategies.
- randomization
- stratification
- patient-treatment matching are some of these techniques.
Randomization:
- The clinical trial techniques has a number of "fundamental" concepts, but none is as inviolable as randomization.
- The CONSORT statement for trial reporting requires the randomization justification, which is a fundamental component of research ethics and trial design courses. There are many main justifications for the need for randomization.
- First of all, it removes selection bias, which can arise from nonrandomized designs even in the most morally dutiful researchers (it is crucial that the researcher and research personnel remain blind to the randomization schedule throughout the trial to minimize selection bias).
- Second, the underlying premise of parametric statistical testing is that participants are assigned to conditions at random.
- Third, it makes it possible to design treatment arms with prognostic variable balance, meaning that the only systematic variations across the arms are related to the intervention.
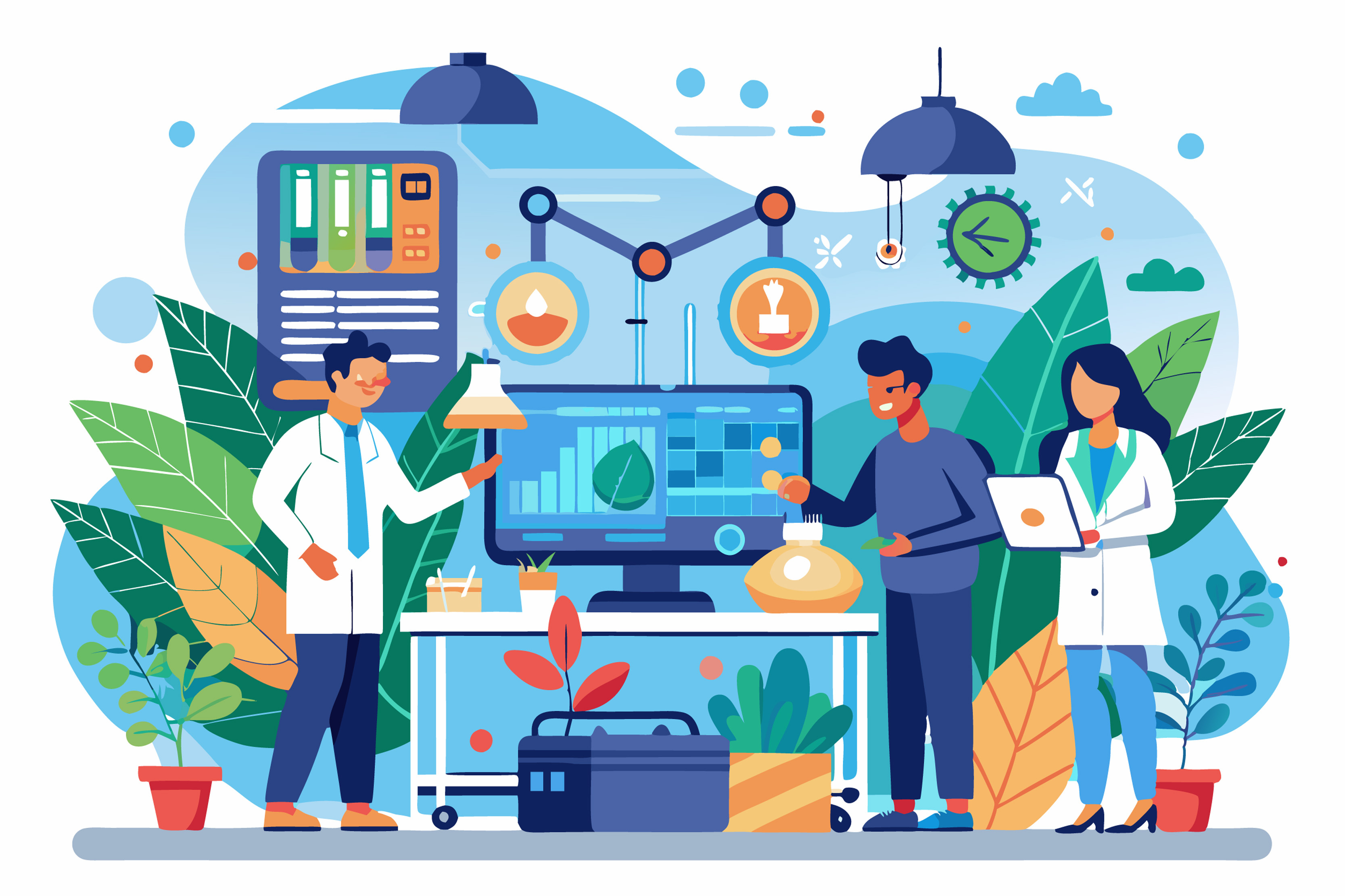
Stratification:
- A random permuted-block design can help with some, but not all, of the possible issues with treatment arm equity in clinical trials.
- Stratification has several advantages. Initially, it guarantees a legitimate comparison between groups, which is particularly helpful in smaller studies with less than 100 participants in each treatment arm.
- It also lowers Type II and Type I errors.
- Thirdly, it reduces the number of stratification variables required to find a significant difference between two groups (boosting power by optimizing between-strata variability while minimizing within-strata variability
- It should be also noted that adding too many stratification variables results in a loss of power due to fewer degrees of freedom in the analysis).
- Fourthly, it shows evidence of prior awareness of prognostic variables.
- Last but not least, stratification enables moderator analysis among treatment moderators that have been previously proposed (groups would be stratified on the hypothesized moderator).
Treatment Matching:
- The idea that individuals with the same ailment or disease category will respond uniformly to any one sort of treatment has been challenged by the increasing attention that heterogeneity of patient samples has received over the past few decades.
- More than 200 patient variables—that is, patient characteristics like coping style, self-efficacy, and locus of control—have been identified as potential sources of treatment matching; of these, more than 100 have undergone empirical scrutiny, and only a small number have been consistently linked to different treatment responses.
For Example,
- For headache medication, we at most have a few number of well-tested clinical pathways or algorithms to help in treatment-patient matching; for behavioral headache therapies, we virtually lack validated treatment-patient algorithms.
- The idea of matching different behavioral therapies to patient subgroups with headache problems has not received much attention.
- The more complex individuals seen in the previously described headache treatment studies are the ones who are most likely to be referred for behavioral management because they are the ones who are more likely to be resistant to either medication or behavioral therapy alone.
The Importance of Patient Stratification in Clinical Trials:
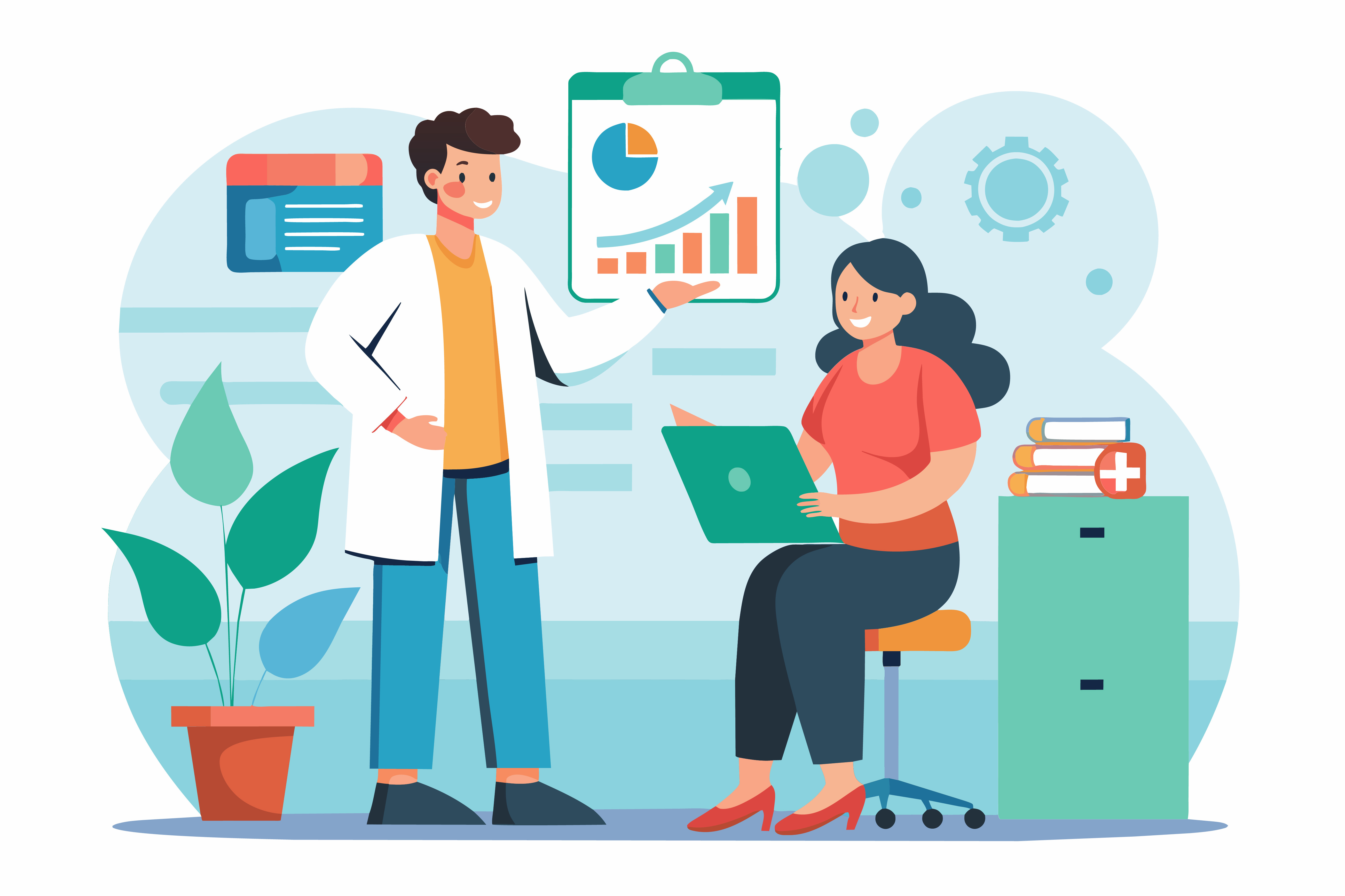
In clinical trials, patient stratification guarantees that medicines are evaluated on the most suitable groups, producing more dependable and significant outcomes. The likelihood that a novel treatment will be shown to be safe and effective can be increased for researchers by identifying and choosing individuals who are most likely to benefit from a given therapy. This focused strategy reduces the risks and expenses related to clinical trials while simultaneously quickening the medication development process.
Clinical Trials Conferences:
Experts emphasize the impact of machine learning (ML) algorithms in transforming patient stratification at the World BI Clinical Trials Innovation Programme. Extensive data from genetic research, clinical databases, and electronic health records may be analyzed by machine learning (ML) techniques like decision trees, neural networks, and clustering to find patterns and correlations that would not be visible with conventional approaches. Through improved patient segmentation made possible by these insights, clinical studies involving the most pertinent patient groups are carried out.