AI in healthcare diagnostics marks a groundbreaking advancement in medical technology, leveraging artificial intelligence to revolutionize disease detection and diagnosis. By swiftly and accurately analyzing extensive patient data, AI algorithms identify anomalies, forecast outcomes, and aid healthcare professionals in making well-informed decisions. This fusion of cutting-edge computing and medical expertise not only enhances diagnostic accuracy but also streamlines processes, cuts costs, and ultimately enhances global patient care.
AI in Healthcare Diagnostics
AI in healthcare diagnostics refers to the application of artificial intelligence (AI) technologies to enhance the detection, diagnosis, and prediction of medical conditions. This involves using machine learning algorithms to analyze large volumes of patient data, such as medical images, genetic information, and clinical records, to identify patterns and anomalies that may not be easily recognizable by human experts. AI in healthcare diagnostics aims to improve accuracy, speed up diagnostic processes, reduce errors, and ultimately optimize patient outcomes through data-driven insights and decision-support tools. World BI is organizing a Digital Health & AI Innovation Summit aimed at broadening your understanding of healthcare and AI.
Importance and Impact of AI in Improving Healthcare Diagnostics
Here are the key points on the importance and impact of AI in improving healthcare diagnostics, simplified and presented clearly:
1. Enhanced Accuracy:
AI can quickly and accurately analyze large amounts of data, aiding in early and precise disease detection.
2. Personalized Treatment:
By analyzing individual patient data, AI helps tailor treatments to specific factors like genetics and lifestyle, improving outcomes.
3. Improved Image Analysis:
AI-powered systems assist radiologists in interpreting medical images like MRIs and X-rays with high accuracy, spotting issues that may be missed.
4. Predictive Insights:
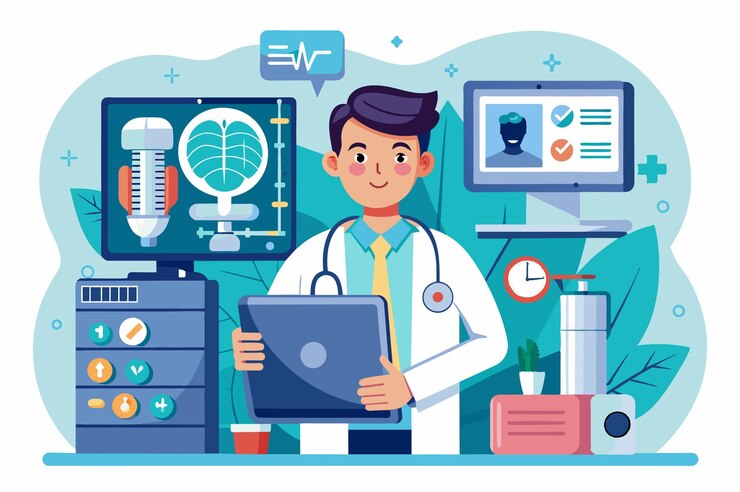
Machine learning predicts disease progression and patient outcomes based on data, enabling proactive healthcare interventions.
5. Efficient Resource Use:
AI optimizes resource allocation, streamlines tasks, and prioritizes patient care, improving operational efficiency.
6. Remote Monitoring:
AI enables real-time monitoring through wearable devices, helping manage chronic conditions and reducing hospital visits.
7. Advancements in Research:
AI accelerates medical research by analyzing data to discover patterns, develop new drugs, and understand diseases better.
Types of AI used in Healthcare Diagnostics
- Machine Learning (ML)
- Natural Language Processing (NLP)
- Computer Vision
- Deep Learning
- Predictive Analytics
- Robotics and Automation
- Virtual Health Assistants
Applications of AI in Healthcare Diagnostics
Here are the applications of AI in healthcare diagnostics presented in a plagiarism-free format:
1. Medical Imaging Analysis:
AI analyzes medical images like X-rays and MRIs to detect abnormalities and assist radiologists in accurate diagnoses.
2. Pathology and Histopathology:
AI aids pathologists in analyzing tissue samples to identify cancerous cells and other anomalies with high precision.
3. Genomics and Personalized Medicine:
AI uses genomic data to predict disease risks and customize treatment plans based on individual genetic profiles.
4. Clinical Decision Support Systems (CDSS):
AI-powered systems integrate patient data and medical knowledge to assist healthcare providers in making informed diagnostic and treatment decisions.
5. Remote Monitoring and Telemedicine:
AI facilitates remote monitoring of patient health through wearable devices, enhancing chronic disease management and reducing hospital visits.
6. Drug Discovery and Development:
AI accelerates drug discovery by analyzing large datasets to identify potential candidates and predict their effectiveness.
7. Patient Risk Prediction and Outcome Forecasting:
AI models predict patient outcomes and disease progression, enabling early intervention and personalized care planning.
8. Healthcare Operations Optimization:
AI optimizes hospital operations by predicting patient admission rates, scheduling staff efficiently, and managing resources effectively.
9. Natural Language Processing (NLP) in Healthcare:
AI-powered NLP extracts insights from clinical documentation to support clinical decision-making and research.
Challenges and Limitations
While AI offers significant potential in healthcare diagnostics, several challenges and limitations must be addressed:
1. Data Quality and Quantity:
AI algorithms require vast amounts of high-quality data for training and validation.
In healthcare, obtaining diverse and well-annotated datasets can be challenging, affecting algorithm accuracy and generalizability.
2. Interpretability and Trust:
AI-driven diagnostic decisions often lack transparency, making it difficult for healthcare providers to understand how algorithms arrive at conclusions.
This lack of interpretability can hinder adoption and trust among clinicians.
3. Regulatory and Ethical Concerns:
Healthcare AI applications must comply with stringent regulations regarding data privacy, security, and ethical use.
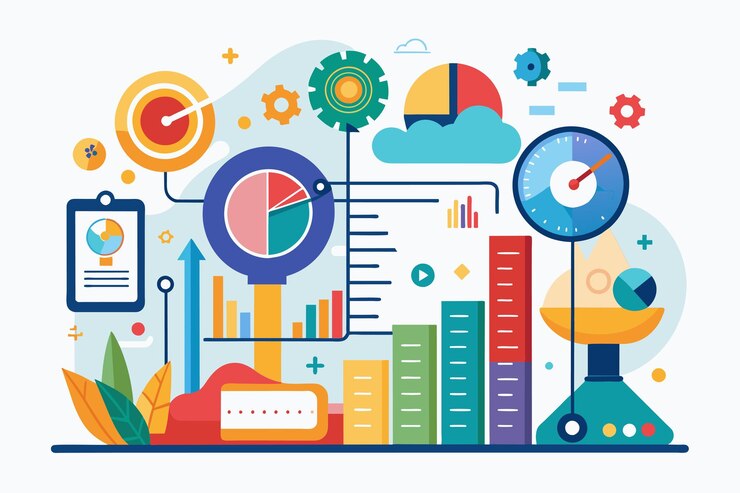
Ensuring algorithms are fair, unbiased, and accountable remains a critical challenge.
4. Integration with Clinical Workflow:
Integrating AI tools into existing clinical workflows seamlessly is complex.
Healthcare systems must ensure interoperability with electronic health records (EHRs) and other IT systems, minimizing disruption to daily operations.
5. Cost and Resource Constraints:
Implementing AI in healthcare requires significant financial investment for infrastructure, training, and maintenance.
Limited resources in healthcare settings can pose barriers to widespread adoption.
6. Validation and Standardization:
AI models need rigorous validation to ensure reliability and consistency across different patient populations and healthcare settings.
Standardizing evaluation metrics and protocols remains a challenge.
7. Human-Machine Collaboration:
Achieving effective collaboration between AI systems and healthcare professionals is essential.
Training clinicians to interpret AI-generated insights and fostering trust in AI recommendations are ongoing challenges.
World BI Digital Health & AI Conference
AI is revolutionizing healthcare diagnostics by significantly improving accuracy, efficiency, and personalized patient care. AI's current applications include advanced medical imaging analysis, pathology, genomic medicine, and clinical decision support systems, all of which enhance diagnostic precision and treatment outcomes. Looking forward, the future of AI in healthcare diagnostics holds immense potential. Continued advancements in deep learning, personalized medicine, and augmented intelligence will further refine AI's capabilities, making healthcare more tailored and effective. Ethical considerations and regulatory frameworks will be crucial in ensuring responsible AI deployment. As AI integrates with IoT and big data, remote monitoring, and proactive health management will become more accessible, transforming healthcare delivery worldwide. Overall, AI's evolving role promises to drive innovation, improve patient outcomes, and shape the future of healthcare diagnostics in profound ways. World BI is organizing a conference on Digital Health & AI Innovation Summit, designed to enhance your knowledge in healthcare and AI. For updates, follow us on the World BI website.