Clinical operations are the foundation of the pharmaceutical and healthcare industries. The process, which includes everything from organizing and carrying out clinical trials to overseeing regulatory compliance, is infamously difficult, costly, and time-consuming. Conversely, artificial intelligence (AI) is emerging as a potent ally in the modern era, providing fresh approaches to persistent problems in clinical operations. World BI is organizing Clinical Trials Innovation Programme again where this topic is going to be discussed.
The Complex Landscape of Clinical Operations
- Recruiting and retaining patients: One of the key challenges in clinical trials is still finding the correct patients, particularly for uncommon or specialized disorders.
- Overwhelming amounts of data: Clinical trials produce vast amounts of data, ranging from patient data to protocol violations, which must be regularly tracked, cleansed, and examined.
- Protocol complexity: As protocols grow more complex, they are subject to more changes, which pushes back deadlines and raises expenses.
- Operational inefficiencies include delays, duplication of effort, and miscommunication caused by manual data entry, disjointed systems, and departmental silos.
- Compliance and safety monitoring: Ensuring that Good Clinical Practice (GCP) is followed and staying up to date with constantly changing regulatory standards is a huge undertaking.
How AI is Addressing These Challenges
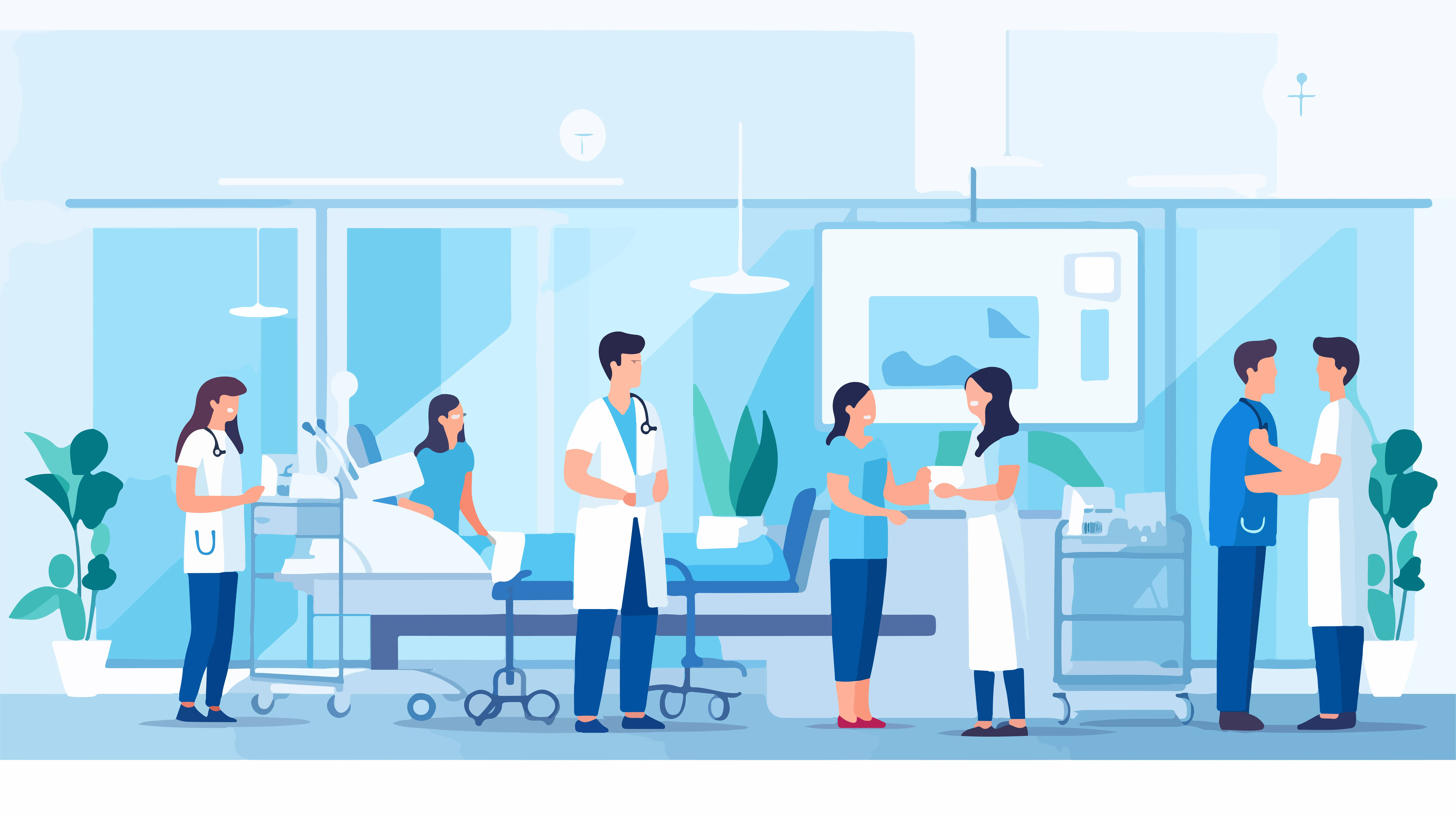
AI technologies, particularly machine learning (ML), natural language processing (NLP), and robotic process automation (RPA), are being applied to clinical operations with remarkable results.
1. Smarter Patient Recruitment
Large datasets, including social media activity, genomic data, and electronic health records (EHRs), can be scanned by AI-powered algorithms to find patients who meet the requirements of clinical trials.
Benefits:
- Speeds up recruitment by narrowing down eligible candidates.
- Reduces screen failure rates.
- Improves diversity and representation in trials.
For example, AI tools can identify patients with rare genetic mutations by analyzing their EHRs across multiple hospitals, a task that would take humans weeks or months.
2. Automating Routine Administrative Tasks
RPA (Robotic Process Automation) can streamline repetitive manual tasks such as scheduling, data entry, and document management.
Benefits:
- Reduces operational burden on clinical staff.
- Minimizes human error.
- Minimizes human error.
This is especially useful for managing site-level documentation, where AI can automatically flag missing fields or outdated documents, ensuring regulatory compliance.
3. Predictive Analytics for Trial Optimization
- Which sites are most likely to enroll on time?
- Which patients are at high risk of dropping out?
- Which protocol deviations are most likely to occur?
Benefits:
- Informed decision-making.
- Reduced trial delays.
- Improved trial design and feasibility assessments.
For example, if a predictive model indicates that a specific site constantly performs poorly, operations teams can act quickly to either reallocate resources or offer more support.
4. Natural Language Processing for Protocol Review and Monitoring
Frequently, protocols are intricate papers with subtle terminology. These documents can be analyzed by NLP, which can also indicate ambiguities or discrepancies by comparing them to previous protocols.
Benefits:
- Reduces the number of costly protocol amendments.
- Accelerates protocol design and review.
- Ensures alignment between stakeholders.
NLP can also analyze investigator notes or adverse event reports in real time, enhancing pharmacovigilance and safety monitoring.
5. Risk-Based Monitoring (RBM) and Real-Time Oversight
AI enables continuous data review, allowing for proactive identification of risks instead of retrospective analysis.
Benefits:
- Enables remote monitoring.
- Reduces the need for on-site visits.
- Enhances patient safety and trial integrity.
AI-enhanced RBM optimizes resource allocation, improves compliance, and concentrates monitoring efforts where they are most required.
Real-World Examples of AI in Clinical Operations
- Pfizer has used AI to enhance protocol design and streamline feasibility assessments, reducing time-to-trial.
- IQVIA uses AI to analyze global site performance and determine optimal trial locations.
- Deep6 AI collaborates with healthcare systems to match patients with clinical trials using EHR mining.
These implementations show that AI isn’t just theoretical. It’s already creating tangible improvements in timelines, costs, and outcomes.
Challenges to AI Adoption in Clinical Operations
Despite the benefits, AI implementation isn’t without challenges:
- Data Privacy: AI models require vast amounts of data to work reliably. Training health care AI may involve feeding it terabytes of medical records.
- Interoperability: Healthcare systems are often fragmented, making it difficult to integrate AI tools across platforms.
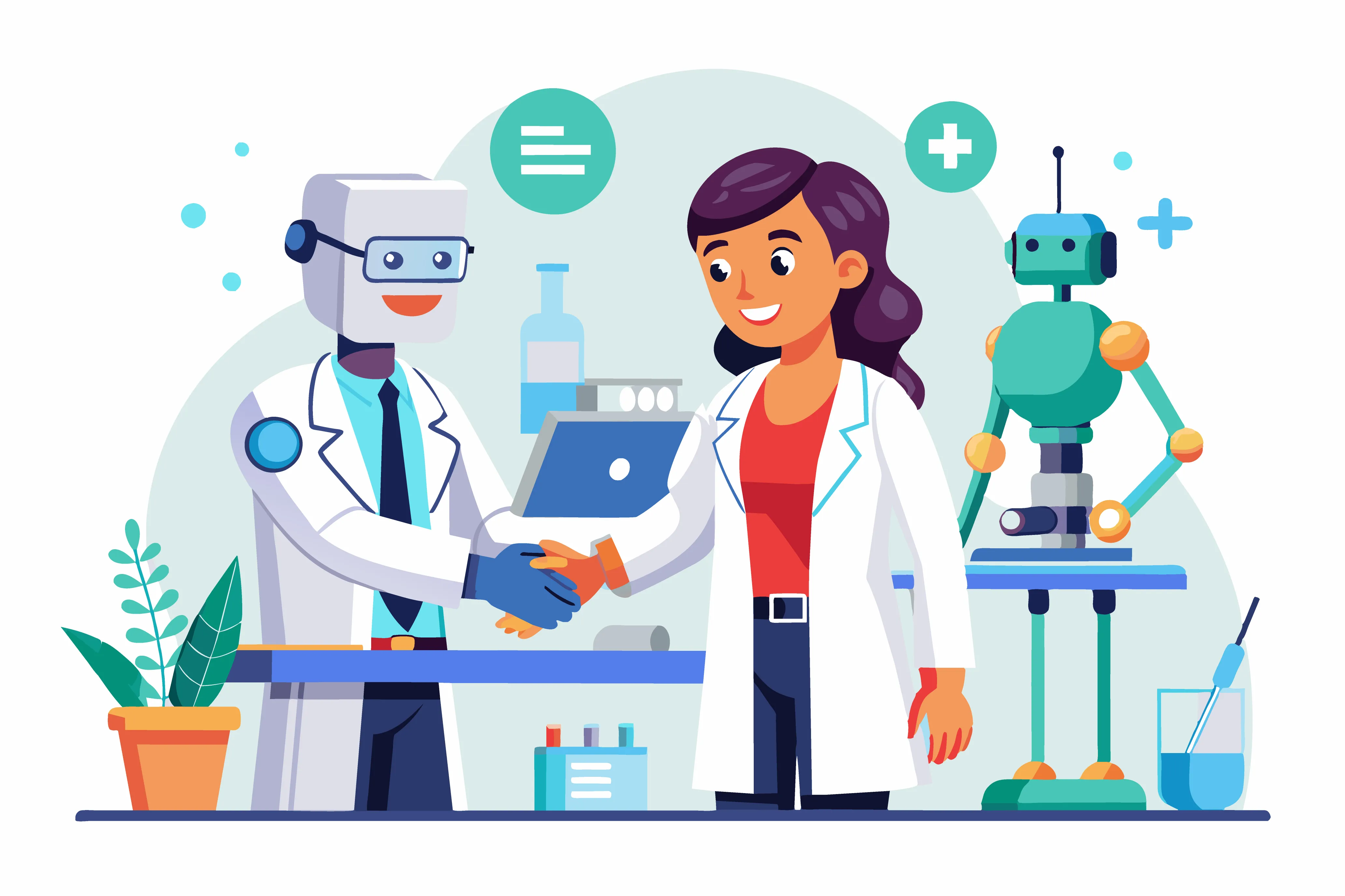
- Trust and Transparency: Stakeholders must understand how AI models work to build confidence in their decisions.
- Change Management: Clinical teams need training and cultural adaptation to trust and adopt AI tools fully.
The Way Ahead
Although it is still in its infancy, the use of AI in clinical operations is expanding quickly. AI's role will only grow in importance as data quality improves and regulatory agencies offer more precise guidelines on its application.
Future Trends to Watch:
- AI-generated synthetic control arms for trials.
- Real-time virtual assistants for site coordinators and CRAs.
- AI integration with decentralized clinical trial platforms.
- AI-driven clinical trial simulations and digital twins.
With the right governance, investment, and collaboration between pharma, regulators, and technology providers, AI will redefine how clinical trials are run from recruitment to reporting.
Conclusion
Clinical operations are ready for change, and artificial intelligence (AI) provides the means to bring about efficiency, insight, and creativity at every stage. AI enables businesses to get better results more quickly, from improving risk monitoring and trial protocol optimization to speeding up patient recruitment. However, success calls for more than simply technology; it also calls for careful integration, support from stakeholders, and a dedication to the moral and responsible application of AI. Future therapeutic progress will be shaped by those who embrace AI now as we traverse this fascinating area.
Clinical Trials Innovation Programme
Clinical Trials Innovation Programme is a global event uniting leading pharmaceutical, biotech, and clinical research organizations along with AI, data science, regulatory, and clinical operations experts to explore the future of clinical development. Clinical Trials Innovation Programme organized by World BI, this dynamic conference focuses on clinical trial design, patient recruitment, decentralized trials, real-world data, regulatory compliance, and AI-driven innovations in clinical research. The event fosters cross-industry collaboration and innovation to enhance the efficiency, diversity, and success of clinical trials globally.