Supply chain planning is vital to the pharmaceutical business, which is heavily regulated and vital to human health. It is not only a question of cost-effectiveness. It takes accuracy, speed, and resiliency to make sure that life-saving medications are produced, shipped, and supplied in the appropriate amount and at the appropriate time. In pharmaceutical supply chain planning, machine learning (ML) is becoming a potent ally as global supply networks get more intricate and patient demand becomes less predictable. By bringing manufacturers and suppliers together, World BI's Pharma Supply Chain & Logistics Innovation Programme helps them identify the best partners to fulfill their pharmaceutical warehousing requirements.
The Significance of Supply Chain Planning in Pharmaceutical supply chains have particular difficulties, in contrast to other industries:
- Strict legal obligations.
- Logistics of the cold chain for medications that are temperature-sensitive.
- Extended and fluctuating cycles of production.
- High risks of overstocking or stockouts.
- Worldwide sourcing and production.
- Regular interruptions (pandemics, shortages of raw materials, etc.).
Pharmaceutical firms want clever, adaptable solutions to address these issues, and machine learning provides just that.
What is Machine Learning?
Within the field of artificial intelligence (AI), machine learning allows computers to learn from data and make better decisions over time without explicit programming. Machine learning algorithms are used in pharmaceutical supply chain planning to analyze both historical and current data in order to generate precise forecasts and facilitate more intelligent planning choices.
Let's look at some important ways that machine learning is changing pharmaceutical supply chain planning.
Demand Forecasting for Drug Products:
For pharmaceutical companies to guarantee that drugs are accessible when and where they are required, particularly for chronic illnesses and seasonal conditions, accurate demand forecasting is essential.
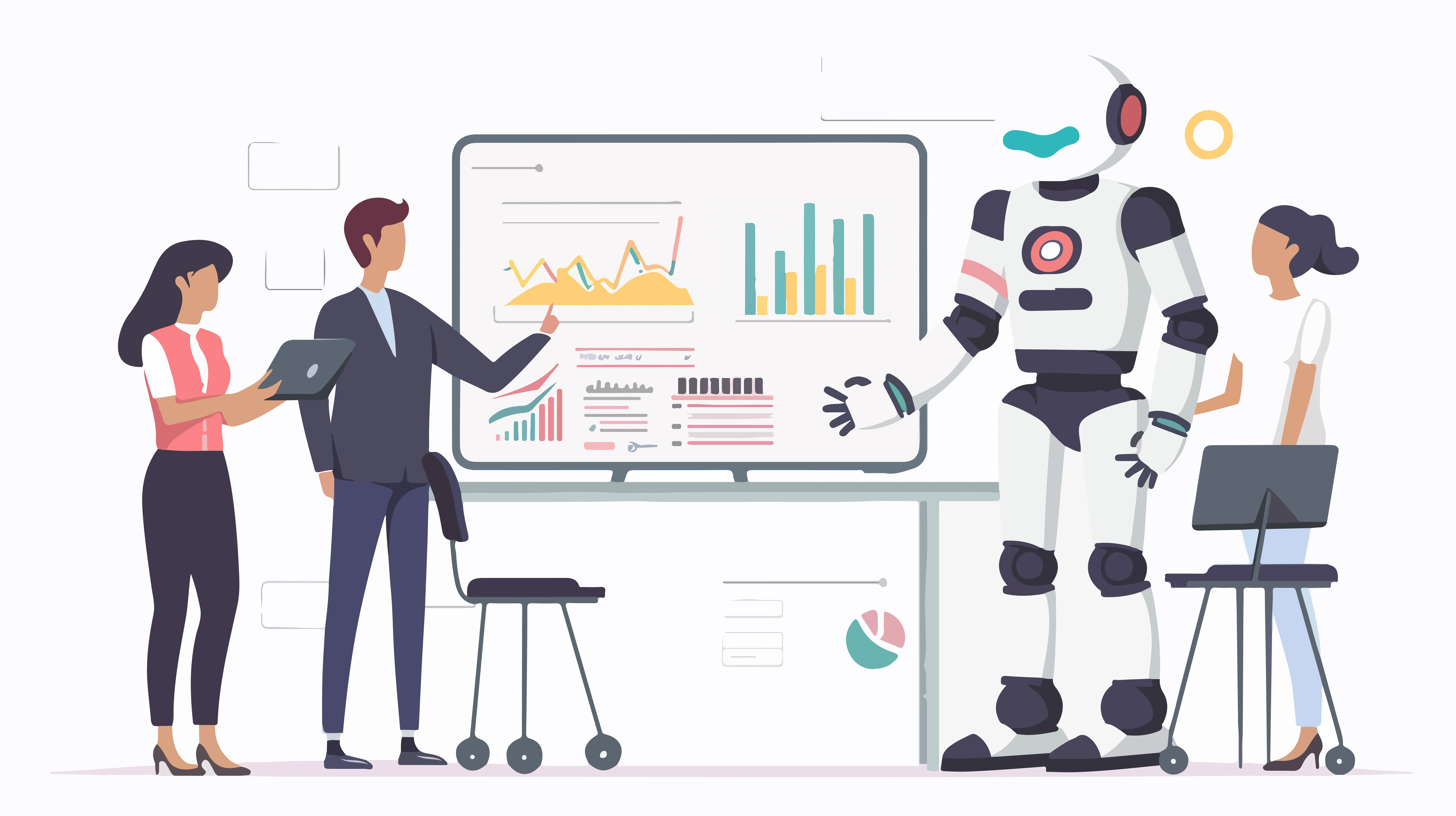
Applications of Machine Learning:
- Examine clinical trial outcomes, prescription patterns, sales statistics, and epidemiological data.
- Forecasts can be dynamically adjusted in reaction to natural catastrophes, pandemics, or changes in regulations.
- Include current information from pharmacies, hospitals, and distributors.
Example: During the COVID-19 pandemic, certain pharmaceutical companies were able to increase production and distribution by using machine learning (ML) to predict surges in demand for vaccinations, PPE, and antivirals.
Inventory Optimization:
Pharmaceutical items need particular storage conditions and may have short shelf life. While stockouts may cause medical delays or even death, overstocking results in waste.
Application of Machine Learning:
- Estimate the ideal safety stock levels for each distribution center.
- Reduce the amount of time-sensitive medications that become obsolete.
- Use real-time demand and lead time data to dynamically modify ordering points.
As an example, a pharmaceutical distributor can use machine learning (ML) to track how often cancer medications are used and send out an automated restocking warning when usage trends show a spike.
Production Scheduling and API Availability:
Manufacturing pharmaceuticals entails extended lead times, intricate procedures, and crucial reliance on active pharmaceutical ingredients (APIs). Production delays might cause the entire timetable to go wrong.
Uses of ML:
- Forecast shortages of raw materials or supplier delays.
- Utilize demand fluctuation to optimize manufacturing cycles and batch sizes.
- Schedule manufacturing across several locations.
In API supply chains, for instance, an ML model might identify risk indicators (such as transportation bottlenecks or geopolitical instability) and suggest alternative sourcing methods.
Distribution and Monitoring of the Cold Chain:
Biologics, insulin, and vaccinations are examples of temperature-sensitive drugs that need cold chain logistics. A minor temperature change can make a medication useless.
- Use data from IoT sensors to forecast and stop temperature fluctuations.
- Reduce transportation time and environmental exposure by optimizing route.
- Examine distribution history to minimize losses and spoiling.
To guarantee compliance, ML models, for instance, might learn from past temperature data collected during transportation and recommend improved carrier routes or packaging choices.
Risk Management for Suppliers:
Pharmaceutical firms depend on a wide range of suppliers for packaging, excipients, APIs, and other supplies. Costly delays may result from supplier interruptions.
- Examine the dependability and performance of suppliers.
- Scan news using natural language processing (NLP) to find early indications of disruption.
- Estimate supply chain risks and suggest ways to reduce them.
An ML model, for instance, can issue a risk alert and recommend substitute suppliers if it finds financial instability in a major supplier based on news reports and market data.
Regulatory Compliance and Serialization:
Pharmaceutical firms have to handle massive amounts of compliance data due to growing international requirements such as the DSCSA and EU FMD that demand serialization and traceability.
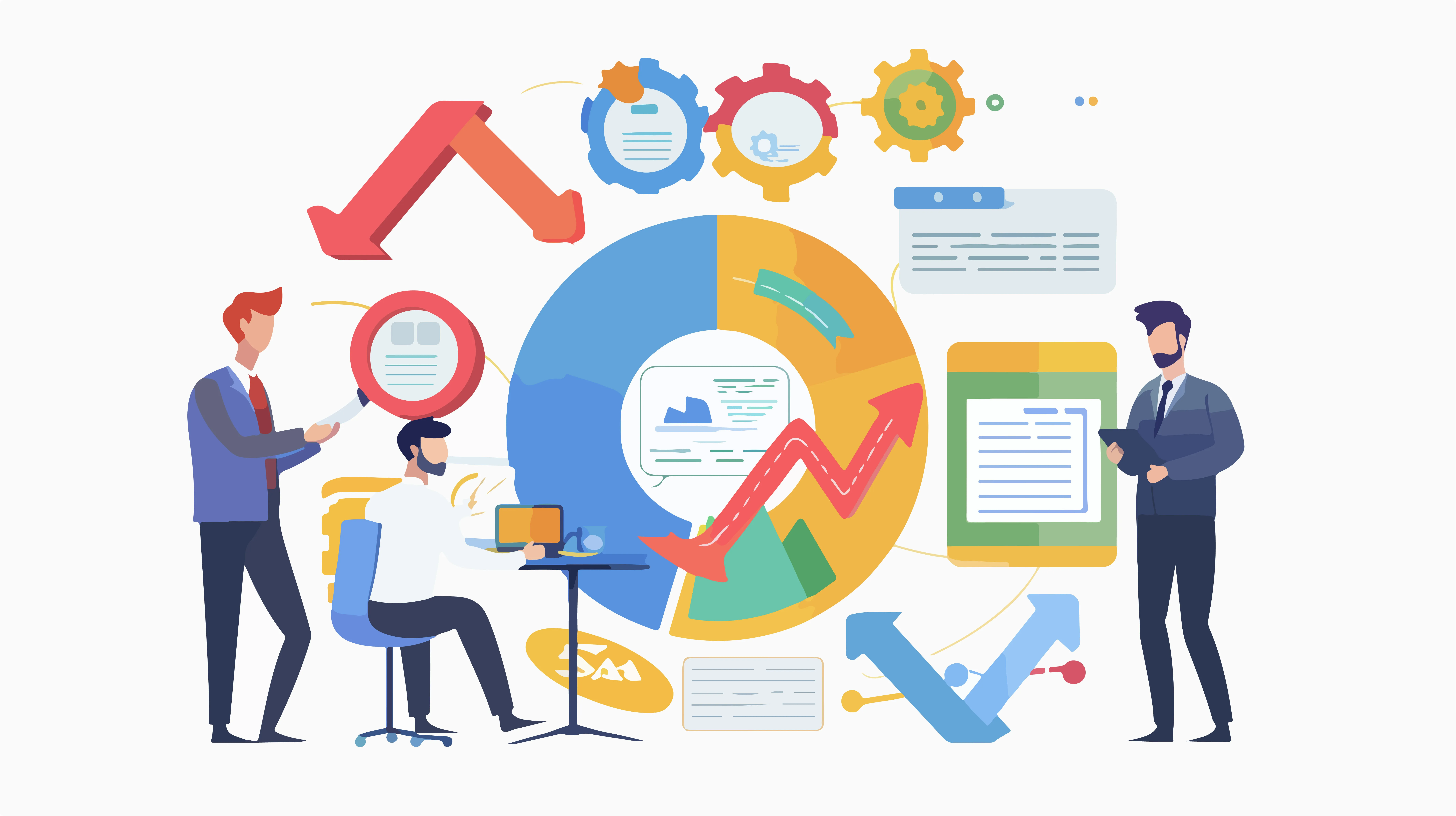
- Detect anomalies in serialization data automatically.
- Keep an eye on compliance throughout the networks of production and distribution.
- Determine trends in non-compliance and suggest remedial measures.
For instance, an ML system may identify anomalous goods movements between marketplaces and designate them as possibly being counterfeit or diverted.
Clinical Supply Chain Planning:
Clinical trial supply networks need careful preparation even before commercial distribution to guarantee that experimental medications are accessible at all trial locations.
- Predict enrollment numbers and medication needs.
- Improve the timeliness of labelling and packing.
- Reduce overproduction and forecast medication waste.
For instance, machine learning (ML) may be used to predict patient enrollment at clinical trial locations and modify medicine distribution schedules appropriately to prevent delays or overstocking.
Benefits of Machine Learning in Pharma Supply Planning:
The following are some advantages of machine learning in pharmaceutical supply planning: increased forecast accuracy, decreased waste and expired inventory, improved regulatory compliance, increased visibility across global supply chains, and quicker reaction to disruptions, effective resource allocation, and higher patient service levels.
Difficulties and Things to Think About:
Despite the obvious advantages, there are certain difficulties in using machine learning to pharmaceutical supply planning:
- Data Silos and Quality: For machine learning to be effective, data must be clean, standardized, and interconnected.
- Legacy Systems: ERP and legacy system integration can be challenging.
- Pharma firms may need to spend money on recruiting or educating ML personnel in order to fill the talent gap.
- Interpretability: Regulatory examination of black-box models may not always be met.
- Change Management: Time and training are needed to make supply chain teams trust and use ML technologies.
World BI Pharma Supply Chain & Logistics Innovation Programme:
The pharmaceutical business is about to enter a new era in which making decisions based on data is not only advantageous but also essential. By facilitating proactive risk management, predictive insights, and more intelligent resource allocation, machine learning provides a revolutionary approach to pharmaceutical supply chain planning. Incorporating machine learning (ML) into supply chain operations will put businesses in a better position to guarantee prompt access to medications, enhance patient outcomes, and create more robust supply networks as the complexity of global healthcare demands increases. Pharma Supply Chain & Logistics Innovation Programme, which provides a platform for distinguished leaders, supply chain professionals, and industry experts from diverse companies to convene. The collective aim is to foster and promote global knowledge within the pharmaceutical logistics sector.
For more information, kindly visit World BI.
We look forward to assisting with all of your supply chain solutions.