AI may greatly improve clinical trials optimization by giving human leaders data-driven insights and suggestions. Organizations may enhance their methods for conducting clinical trials and tackle difficulties in trials design and planning thanks to this cooperative process, which signifies a significant shift in clinical development. AI strengthens human leaders, but it shouldn't be used as a substitute for human decision-making. Through the Clinical Trials Innovation Programme, World BI offers chances to use AI technologies that can facilitate the advancement of clinical trials.
Barriers and Their Solutions
Data Accessibility and Quality
- AI's potential in clinical trials data is limited due to inconsistent, fragmented, and lacking data from various countries, systems, and organizations.
- This can lead to biased or erroneous conclusions in AI models, reducing their usefulness and compromising data accessibility and quality.
Solution: Improved Data Integration and Standardization
- The industry should prioritize data integration and standards to ensure AI models function effectively.
- Addressing data fragmentation issues with standard data formats and interoperable technologies can improve data sharing.
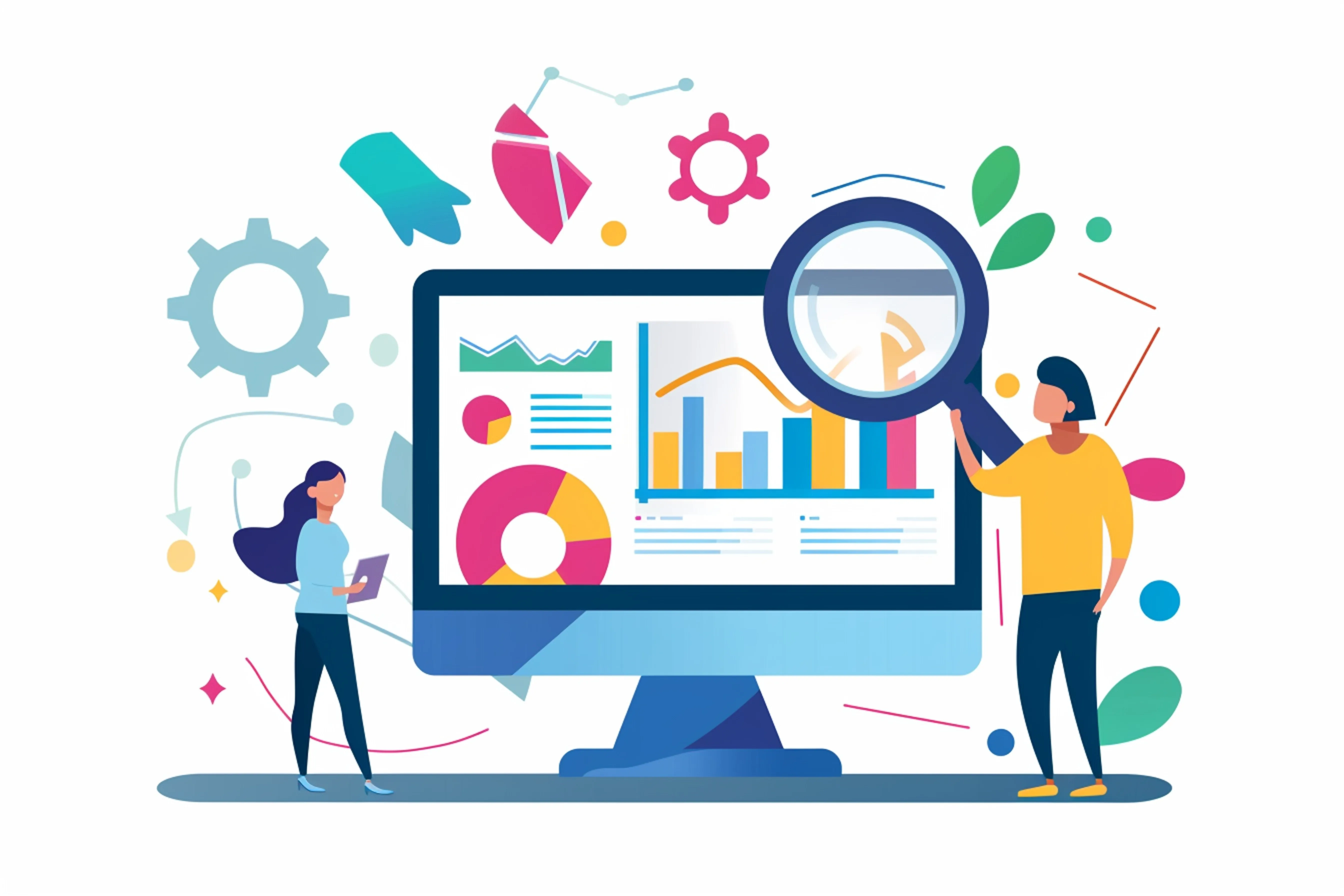
- Implementing "Findable, Accessible, Interoperable, and Reusable" principles can enhance data sharing and provide high-quality datasets for AI systems.
- Maintaining data security and privacy through strong encryption techniques can foster confidence and promote private information exchange.
Regulatory Obstacles: Handling Complicated Regulations
- The evolving regulatory environment poses a significant challenge to the use of AI in clinical trials.
- The regulations like the FDA and EMA are developing unclear rules, leading stakeholders to be cautious due to concerns about potential delays or compliance issues.
Solution: Early Engagement and Cooperation with Regulators
- Pharmaceutical companies and AI developers should collaborate with regulatory bodies early to ensure adherence to changing regulations.
- The FDA has issued guidelines for AI in medical devices and is expanding them to include AI in clinical trials.
- Stakeholders can ensure AI solutions meet safety and effectiveness requirements by collaborating with regulators throughout the development stage.
- Thus engaged in developing adaptable, iterative regulatory frameworks.
Ethical and Bias Issues: Maintaining Equity
- AI models are only as effective as the data they are trained on, and biased data can lead to inaccurate predictions.
- This can result in unrepresentative patient populations, incorrect diagnoses, and potentially harmful therapies during clinical trials.
- Ethical concerns around patient consent and data privacy also arise.
Solution: Continuous Monitoring and Ethical AI Development
- To promote fairness and transparency, developers should implement "explainable AI" (XAI) strategies and diversify clinical trials recruitment.
- Additionally, AI systems should be trained on diverse datasets to reduce bias and improve accuracy.
- Ethical issues should be considered, including patient informed consent, transparent data use, and continuous monitoring for potential ethical or medical repercussions.
- This will help reduce bias and ensure treatments work for different groups.
Insufficient Trust and Comprehension: Teaching Stakeholders
- AI's potential is promising, but there is a lack of trust and understanding among stakeholders, including clinical researchers, regulators, and patients.
- Concerns about accuracy and dependability can make it difficult for physicians to trust decisions made by systems they don't fully understand.
Solution: Education, Training, and Collaboration
- Cooperation, education, and training are crucial for fostering confidence in AI in healthcare.
- Healthcare practitioners must understand its advantages, limitations, and potential. Offering clinical trials seminar and training materials helps demystify AI.
- Tighter ties between experts and AI developers ensure real-world clinical requirements are met.
- The medical community is more likely to accept AI as an additional tool supporting human decision-making.
Resource Restraints: Overcoming Financial and Technical Barriers
- AI in clinical trials can be costly, especially for smaller businesses or those with limited finances.
- The expenses in infrastructure, personnel, and technology can be too heavy for many organizations, especially in public and academic sectors.
AI-as-a-Service and Strategic Alliances as a Solution
- AI-as-a-service platforms offer cutting-edge AI technology without large upfront infrastructure costs.
- Organizations can acquire tools and resources by collaborating with vendors or joining consortia.
- Non-profits, governments, and venture capitalists can support AI-powered clinical studies by offering grants, funding, or incentives.
- This financial assistance helps make the technology more accessible to a broader range of organizations.
Strategies:
Ensuring Diversity and Reducing Algorithmic Bias in AI for Clinical Trials
- Researchers can reduce algorithmic bias by gathering diverse data sets, focusing on underrepresented groups in clinical trials, and developing uniform eligibility requirements.
- This will ensure AI models are trained on diverse patient experiences and improve patient matching automation and recruiting efficiency.
Finding and Employing Data Scientists and AI Specialists
- Successful AI adoption depends on finding skilled workers with data science and AI experience.
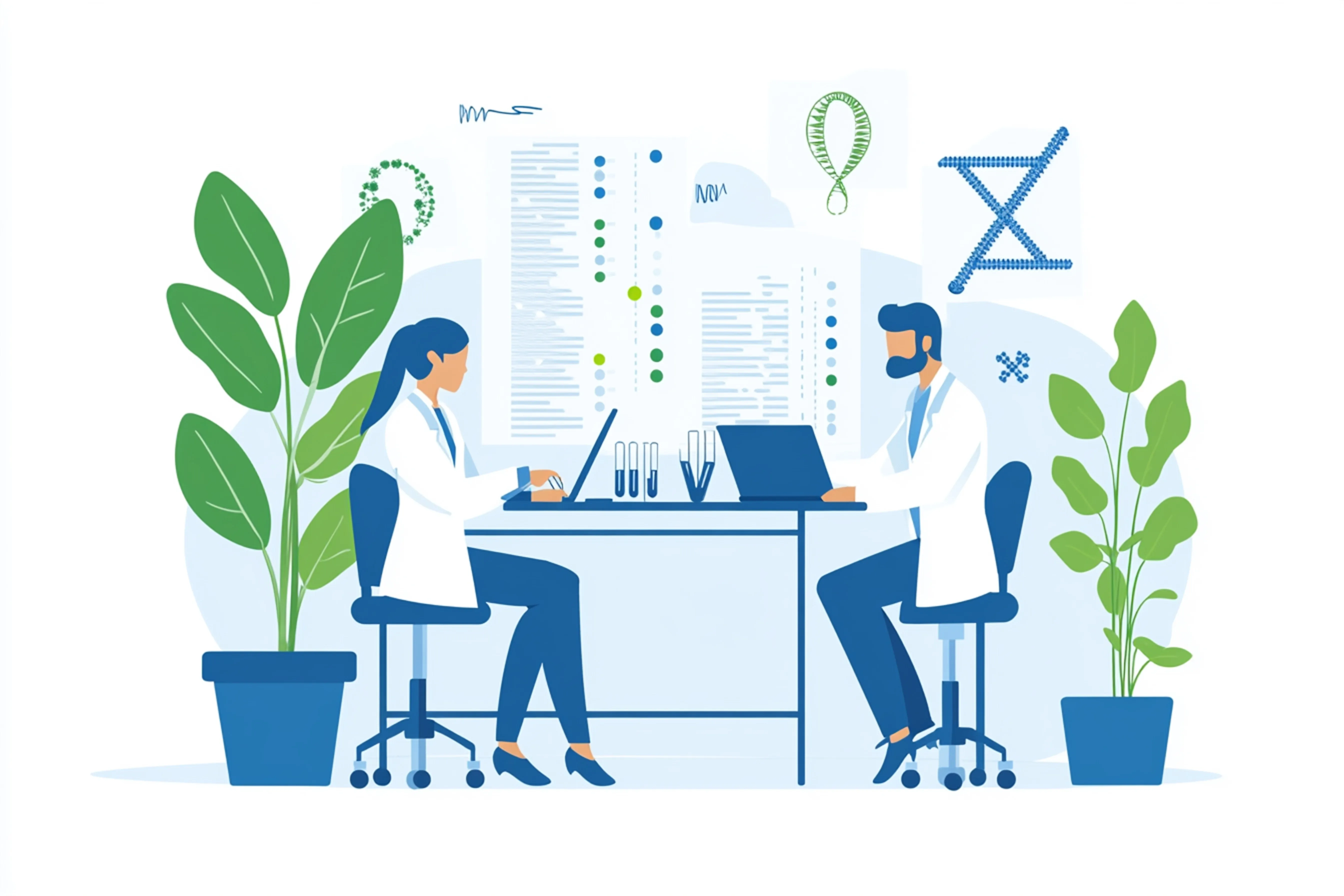
- Companies should aggressively seek out and draw in qualified workers who can spearhead AI projects and help create and execute AI models and solutions.
Leveraging Natural Language Processing (NLP) for Enhanced Data Utilization in Clinical Trials
- AI techniques like natural language processing (NLP) can help process unstructured data, extract relevant information from clinical notes, and facilitate analysis and clinical trials use.
- Cooperation between data scientists, physicians, and patients is crucial for successful AI use, ensuring end users' consideration and usability.
Continuous Monitoring
- Institutions should create regulatory frameworks to oversee the use of AI in clinical trials, ensuring adherence to ethical standards.
- Regular assessments for bias and accuracy must also be conducted to maintain the integrity of AI applications.
Standardizing Data Formats
- Data availability may be increased by standardizing data formats and promoting data exchange between organizations.
- Encouraging the adoption of AI-friendly clinical trials management systems (CTMS) and electronic health records (EHR) can help expedite data access and enhance its quality.
Gradual Integration and Change Management
- Integrating AI technologies gradually and methodically can reduce disruption.
- Teams should start with pilot projects or testing stages to ensure efficacy.
- Change management techniques can ensure smooth adoption.
- AI solutions should be adaptable to blend with current systems, reducing technological obstacles and facilitating a smoother transition.
Conclusion: An AI-Powered Future
AI has the potential to significantly improve clinical trials through improved patient recruitment, lower trials costs, and quicker medication development. However, to fully realize its potential, adoption hurdles must be removed. Resolving regulatory issues, enhancing data quality, ensuring equity, fostering trust, and making AI accessible to everyone can create favorable conditions for AI-driven trials. Cooperation to address these obstacles can ensure AI becomes a useful tool for novel therapies and improved patient outcomes.
Clinical Trials Innovation Programme
To guarantee that AI technologies are applied efficiently and morally, stakeholder collaboration and ongoing monitoring will be essential, opening the door to a new age of clinical research that benefits all patients. Our strategy for expediting the use of AI in clinical trials has resulted in the creation of the Clinical Trials Innovation Programme, which is overseen by World BI. Different biotech businesses may concentrate on exploring new advancements for easier adoption of AI thanks to this innovative process. Companies may save a significant amount of time and money throughout their clinical trials journey by collaborating with appropriate AI service providers.